Predicting how a crisis unfolds
Using artificial intelligence in the humanitarian sector has become increasingly popular. Here’s how a predictive form of the technology works and why it could change how the world responds in the aftermath of a crisis.For Rana Novack, a program leader at IBM, everything began with an orthodontist’s appointment.
Not her own, but her 15-year-old cousin’s, nearly 10,000 kilometres away in Damascus, Syria. It was 2013, two years into the country’s civil war, and violence was creeping closer to the city where the majority of Novack’s family still lived. The internet would cut out intermittently and keeping in touch with loved ones became sporadic. Late one night that year, from her home in northern Virginia, Novack called her family to beg them to leave.
It would be two years before Novack’s aunt and her family would leave Syria, along with many of Novack’s other relatives. During that time, she began to wonder: what might happen if data scientists could predict a refugee crisis before it occurred? Why would people leave, how many would flee and where would they go? Novack hypothesized that if governments knew a crisis was coming far enough in advance, they might be better prepared to receive refugees and coordinate a global response. That preparation could open up more opportunities to leave, opportunities her family could have taken before the violence escalated. The idea led Novack and her colleagues at IBM to successfully develop such a tool – a version of which is planned to make real-life predictions later this year.
Behind a prediction
It all starts with predictive analytics, a statistical technique that uses existing data, machine learning and artificial intelligence to generate predictions about future events. The technique involves analyzing data and running it through algorithms – a set of rules that tells a computer what to do – to create a model. Current data is then fed into the model, which results in a prediction. It’s a form of technology that people interact with every day. Predictive analytics is how variables like humidity and wind direction are used to forecast the weather, how Amazon and Netflix can tell you what you should buy or watch next or how you’re assigned a credit score to determine whether or not you can take out a loan. Companies like Microsoft and IBM have long experimented with the technology, primarily for commercial purposes. Most recently, IBM achieved 95 per cent accuracy in identifying employees thinking of quitting a company so bosses could retain them before they decided to leave.
In the past several years, tech companies and aid organizations began to realize that the technique could also be applied to the humanitarian sector. This paved the way for new programs like Microsoft’s AI for Humanitarian Action, a five-year, $40 million initiative that launched in September 2018. Already, the company says it is using predictive analytics to isolate future famines and disrupt human trafficking networks.
There are some clear benefits to predicting massive events before they occur. If a natural disaster was predicted months before it happened, for example, governments and organizations could be proactive instead of reactive, potentially slashing death tolls and mitigating costly damage. Microsoft’s famine model, developed in partnership with the World Bank and a number of other organizations, is hoping to identify food crises that could turn into full-blown famines. The idea is that exposing a crisis before it escalates can save lives and reduce the amount of money spent on aid.
A similar goal lies behind forecasting a refugee crisis. In one of the first projects to tackle the issue, IBM joined forces with the Danish Refugee Council (DRC) in 2017, a nonprofit humanitarian organization based out of Copenhagen. Together, their teams are working on a project called Mixed Migration Foresight, or MM4Sight, which is using predictive analytics to determine why displaced people move and where they are likely to go. The intent is not necessarily to stave off a crisis, but to predict how it will unfold to help policymakers organize a response.
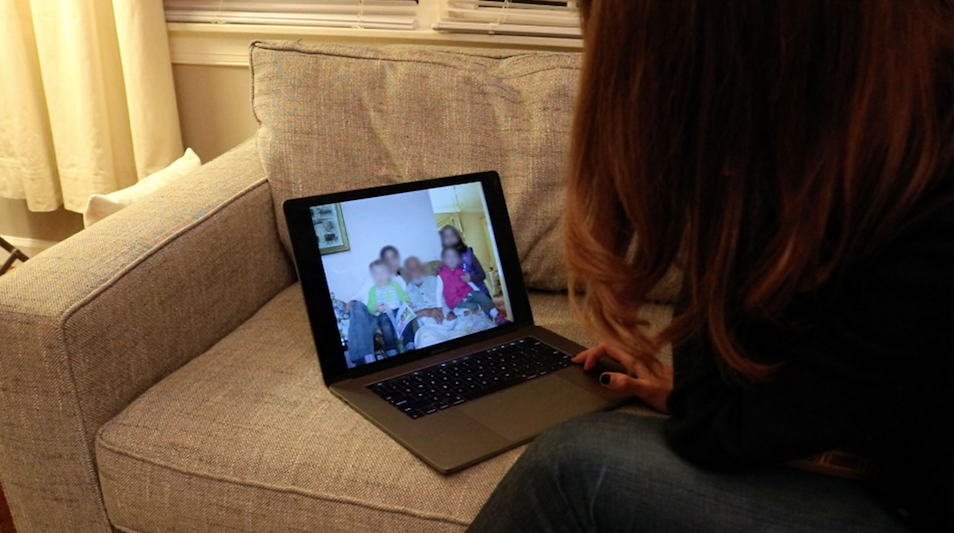
Novack flips through some of the few photos she has of her family in Syria. Though most of them have since left Damascus, she asked that their identities remain protected to ensure their safety. [Photo © Raisa Patel]
To get to that point, IBM needed to start experimenting. In 2016, a group of researchers completed the first step: a prototype of a tool that could predict the migration of refugees along a Balkan route spanning Greece to Austria. The tool couldn’t predict a crisis, but it could predict the movements arising from one. Researchers fed a number of indicators into several models, including the UN High Commissioner for Refugees’ registration data and information about policy changes (such as border closures) from news reports. Even weather data like wind speed was included in the model, because it was found that refugees were less likely to migrate in open water on a windy day.
“For sea-faring refugees arriving in the Greek islands,” a research paper on the prototype noted, “factors such as wind speed were found to be negatively correlated with arrivals over the winter months.”
But not all factors were successful at predicting refugee migration, said Rahul Nair, one of the leading researchers at IBM’s lab in Dublin, Ireland involved with the project.
“Sometimes you’ll have an intuition that something should work and it doesn’t.”
– Rahul Nair
“Sometimes you’ll have an intuition that something should work and it doesn’t,” he said. “An example of that is social media.”
Nair and his colleagues studied a sample of Twitter users and hypothesized that “if there is distress at a societal level, the volume of tweets should be correlated with that.” The team thought that refugees might take to social media to describe their circumstances. But they found that between posts being written in different languages and posts containing irrelevant information, there was too much noise. It was left out of the model.
After the relevant factors for the model were selected, the team had to verify whether their findings would be correct. To do that, data scientists turned to historical data.
“Let’s say you have 20 years worth of data. What you would do is, you would hold five years’ worth of data and you would hide it from the system,” Nair said.
“You would train it on the 15 years of data that you have and then try to predict the values for the period that you hid from the system, knowing fully well what the actual answer was.”
After using historical data from October 2015 to January 2016, the team successfully predicted migration volumes at camps along the Balkan route three weeks in advance. The numbers were close to the volumes that had actually been recorded at that point in time. The tool’s success meant that in the real world, it would be possible to predict where refugees or other displaced people would go weeks before they arrived and prepare accordingly.
When the DRC came on board a year later, the data had to be adapted for the specific scenarios they wanted to test: the movement of displaced people out of Ethiopia. Gone were factors like wind speed and in came more relevant indicators like livestock production.
“If there is a drop in livestock productivity, then it suggests some other systemic factors around food security,” explained Nair. “And food security is related to the propensity of people to move.”
Other important factors were less obvious, like access to tuberculosis treatment.
“Tuberculosis coverage also gives some signal in migration, but that’s likely not because it’s a cause, but more that it’s a proxy for the public health system situation in the country,” said Bo Schwartz Madsen, a DRC data scientist working on the project.
The DRC was able to determine that displaced people were not necessarily leaving an area because of a lack of treatment, but rather because the quality of health care was poor. The findings are evidence that a complex interaction of factors must be uncovered to create an accurate predictive tool.
Work on the first phase of DRC’s migration forecast wrapped up in late 2018. According to Schwartz Madsen, the team now has “some skill” at predicting movements of displaced people out of a country one year in advance. By “skill”, Schwartz Madsen means the model is more accurate than simply “naively guessing” at a value, such as assuming the number of people leaving Ethiopia and heading in a certain direction one year will be the same for the next.
Looking forward
In phase two set to launch later this year, the team hopes to tighten this accuracy, better isolate the drivers behind migration and expand the model to more countries. These will likely be in the Horn of Africa, currently one of the world’s top regions of origin of refugees.
“We do not know what the drivers are with big certainty, and that is quite surprising for some,” Schwartz Madsen said. “We cannot just rely on what people think are the drivers, even though we could maybe get away with it. We have to go and do the actual research.”
IBM and the Danish Refugee Council aren’t the only
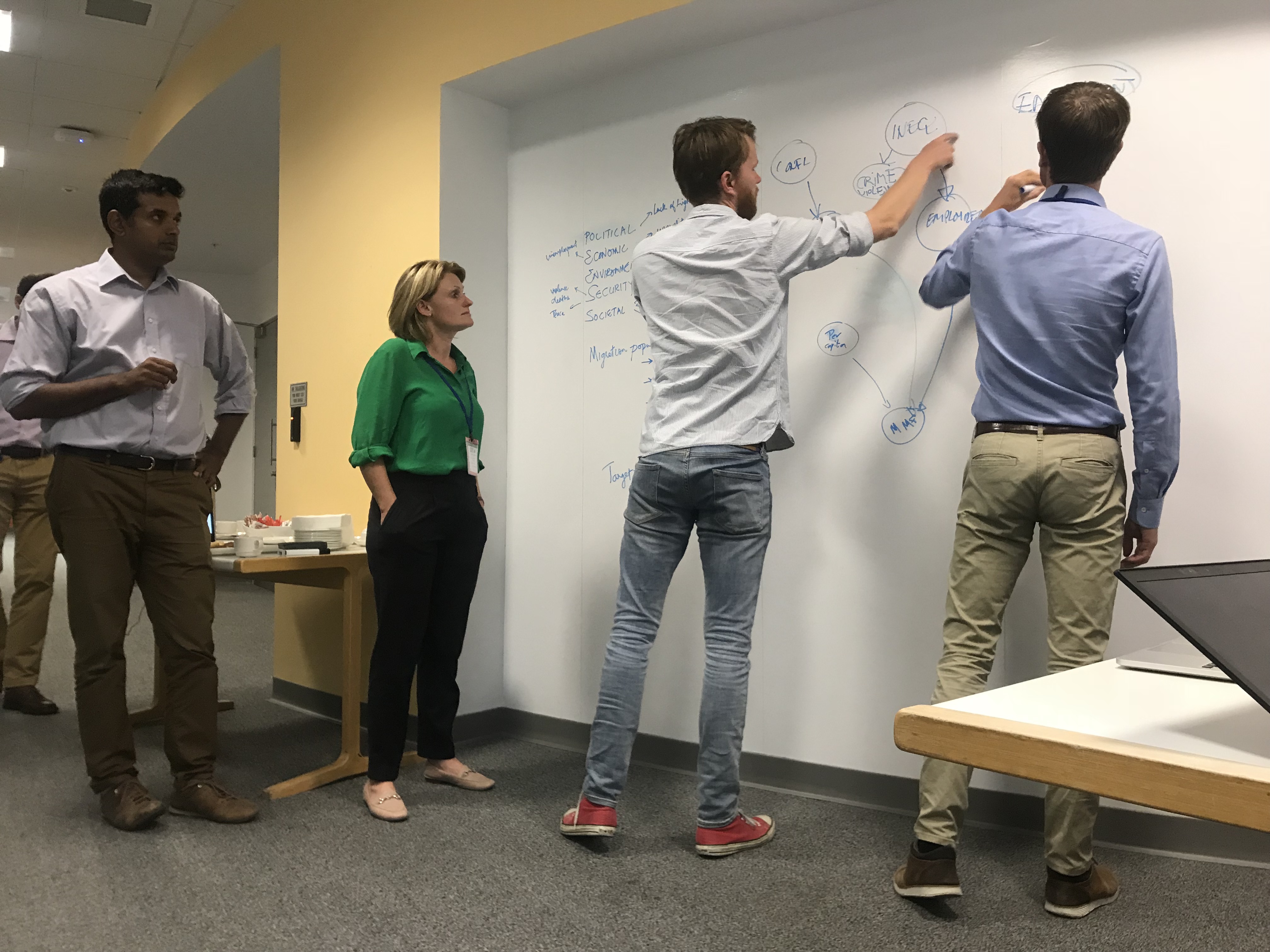
The DRC and IBM teams came together in Dublin, Ireland for a detailed project workshop in August 2018. From left to right: IBM researcher Rahul Nair, DRC original project lead Helena Lassen, DRC data scientist Bo Schwartz Madsen and DRC Mixed Migration Centre lead Bram Frouws. [Photo courtesy of Sanne Urbak]
ones trying to predict refugee movements before they happen – both Microsoft and the UNHCR have similar projects in the works. While Microsoft said it was too early in development to comment on its AI for Humanitarian Action program, according to the company’s website, one of its priority populations is refugees and displaced people.
It hopes to use AI to change “the way frontline relief organizations anticipate, predict and better target response efforts” in areas including famine, human trafficking and providing refugee aid. In an emailed statement, the company said it was “engaging nonprofits across the globe” to carry out the projects.
Given the scope of its data collection, it’s not surprising that the UNHCR also launched its own predictive analytics tool. Known as Jetson, it’s a project the organization is working on independently, though Novack said she’s presented her work to the United Nations in the past. Like IBM’s tool, Jetson looks at the variables that influence refugee migration – like the number of violent conflicts in a region or the level of rainfall – to predict how people will move. And like MM4Sight, Jetson is particularly focused on movements in the Horn of Africa and has been used to predict arrivals in Somalia one month in advance.
A major assumption underlying these projects, however, is that policymakers will see value in such tools. Sometimes, Novack is approached by those who appear skeptical.
“A question I am often asked is, ‘Would we have responded to a refugee crisis any differently if we had seen it coming?’” Novack said.
Her personal answer is a hopeful affirmative, but she is conscious of rising anti-refugee and anti-immigrant sentiment around the world. For tools like MM4Sight, such attitudes could pose a significant barrier to the funding and implementation of predictive modelling in the crisis sphere.
While the point of these tools is to create a more informed – and less expensive – response to crisis events, governments may nonetheless choose not to use them. The United States, for example, capped refugee admissions at record lows in the past two years. It’s a sign that such information could be met with disinterest – or something else entirely. Knowing about a crisis in advance could give a government more time to prevent refugees from crossing its borders. And the possibility exists that the very regimes that produce refugees could use such technology to block avenues of escape.
For now, however, conversations are turning elsewhere as forms of artificial intelligence are becoming more publicly known and widely used. And many of them are about the risks that this technology could impose on vulnerable populations – including refugees.
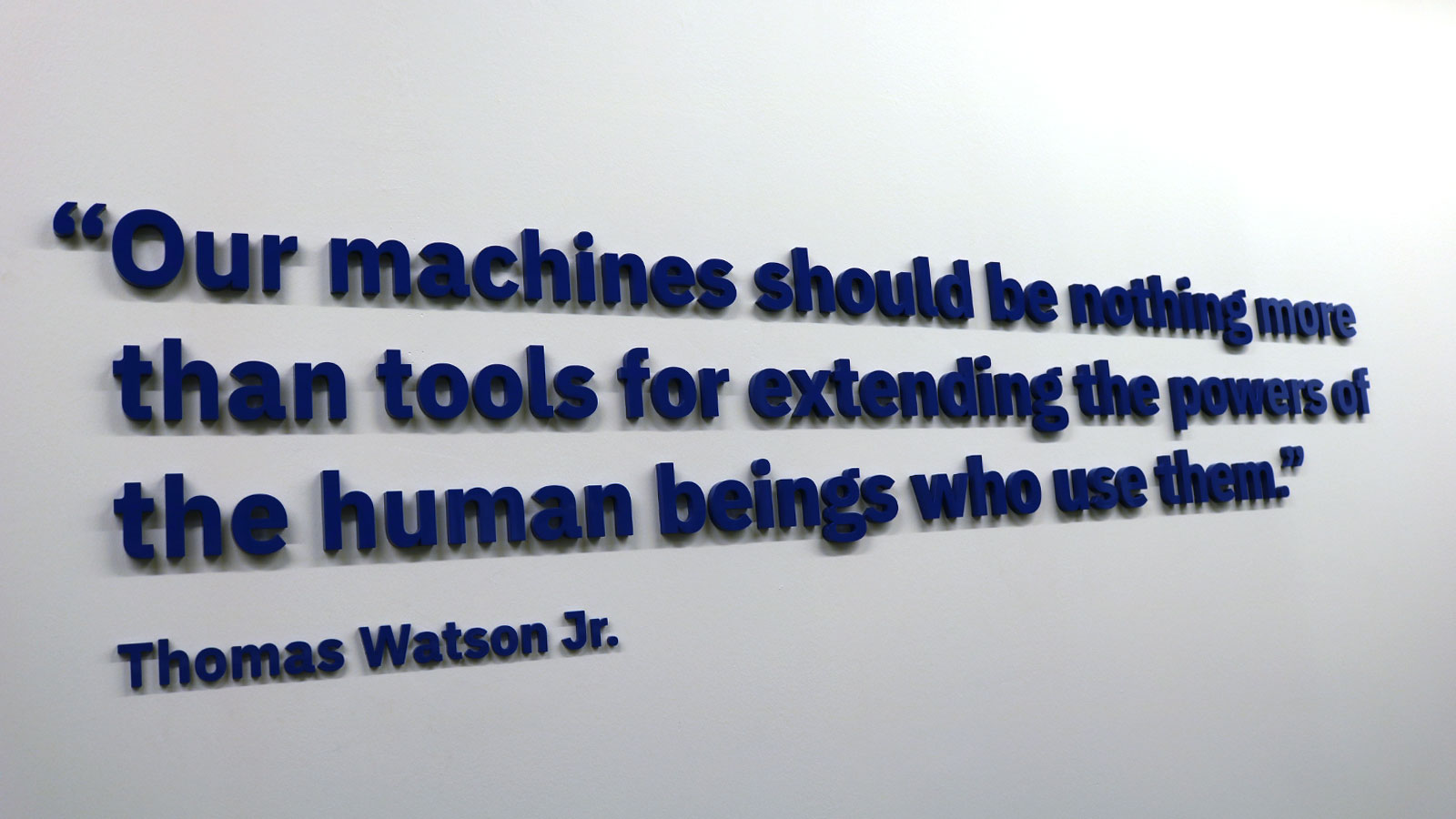
A quote mounted on the wall of IBM’s Washington, D.C. office, said by Thomas Watson Jr., son of the company’s founder. For Novack, it’s a phrase that warns just as much as it inspires. [Photo © Raisa Patel]